On this episode of Rise of the Next, Christina Cai talks about how her company Lydia.ai applies non-traditional metrics to help insurers underwrite health plans.
2022.10.14
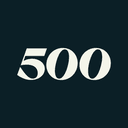
500 Global Team
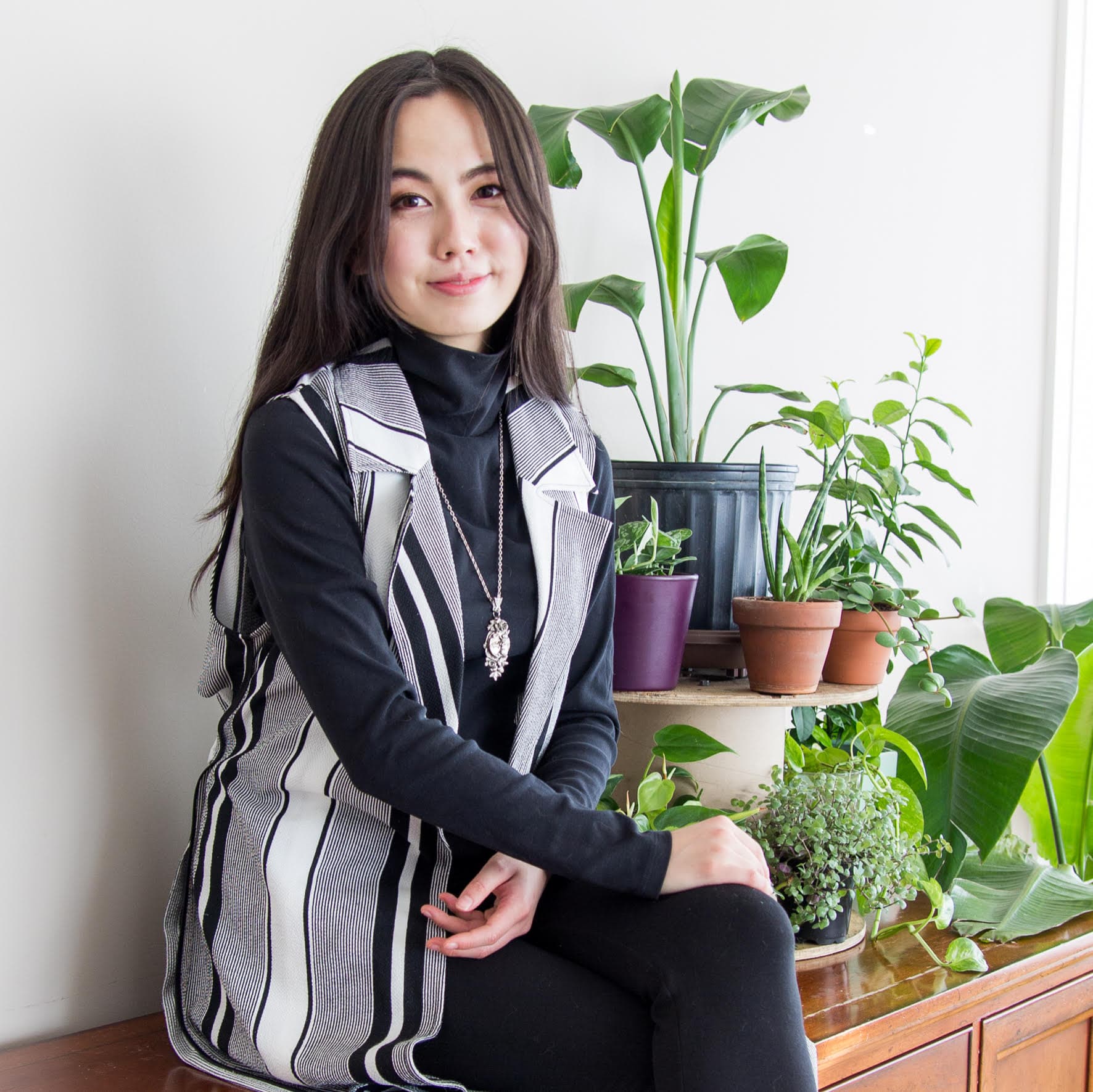
Picture courtesy of Lydia.ai
A techie, Christina Cai stumbled into healthcare when she realized that her AI-enabled technology could unlock insights into previously unused data in electronic health records, wearables and other digital health tools, such as glucose and blood pressure monitors. That information could provide a different assessment of a person’s health risk, and potentially lower the barrier to getting health insurance. As an example, she says people who have diabetes but maintain a healthy lifestyle, can have a more positive health assessment score than a sedentary person.
Lydia.ai now operates in Taiwan, where the government maintains a national health database that is accessible to third party developers, with patient consent.
Christina shares these insights and more on Rise of the Next.
Subscribe here to episodes of Rise of the Next on major streaming platforms.
TRANSCRIPT
Shereen Abdulla
Christina, welcome to Rise of the Next. It’s wonderful to have you on. I’d love to start by asking you to tell us about yourself. What is Lydia AI, and how did you come up with the idea?
Christina Cai
Thank you for having me. My name is Christina, I’m the Co-founder and CEO at Lydia AI. We help life and health insurance companies figure out how to protect more people by using new sources of data to underwrite them. The way it works is that we look at different data sources such as your wearable, your sleep, your steps, your accelerometer, your medical records, and other lifestyle factors, and we actually use that to create a health index that actually mimics your biological age. So that’s your actual health, as opposed to your health risk based on your age. Then, from then on, we give consumers a better understanding of how their health is evolving and how their health compares to people in their age and gender group. We give insurance companies the ability to use these new sources of data that actually predict how a person’s health risk, compared to somebody in their health and age and gender groups, we allow them to instantly and accurately assess their health risks so that they can actually offer them personalized policies and actually figure out how to insure more people.
So, when we first started, our original — we were just a machine learning company — was actually, make the world’s healthcare data useful. Because there was so much healthcare data (most of it is in freeform text), it was unused. We had a piece of technology that allowed us to structure it so that it can be fed into machine learning models. And so, we had all this ability to unlock previously unused data, spit out predictions, and it took us a while to be like, “Hey, you know what, the industry that could most benefit from a better way to index and assess health risks was actually life and health Insurance.” Then that’s how we ended up in the life and health insurance industry.
Shereen Abdulla
What problem are you trying to solve?
Christina Cai
So, when we really look at it, life and health insurance in emerging regions struggle with innovating products, because everybody’s using the same country-level disease incidence. Disease rates are based on — okay, this is how old you are and your gender. And really, a lot of the times people don’t necessarily fit into these neat, little boxes, especially when it comes to categorizing their health. So, what ends up happening is that insurance companies, first of all struggle to innovate with great insurance products that fit their needs, second of all they struggle with underwriting a lot of these people at the correct and accurate risk that they actually have. So, they end up missing a lot of people. A lot of people end up falling through the cracks, getting rejected. And, third of all, for customers, there’s a huge barrier to actually purchasing it.
Shereen Abdulla
And why does this problem exist in emerging regions specifically?
Christina Cai
I think when, we look at it, this issue is all over the world. In every country, you can find — you will find that people have gone through medical bankruptcy. And the reason behind that is because, despite universal health care coverage, it’s usually coverage for universal basic coverage. So there’s still a portion of it that is actually out-of-pocket costs. So, if you’ve received a medical bill, and you’ve had to pay some sort of amount out-of-pocket, then you understand that this portion that you have to pay yourself is either covered by a private health insurance, or by your savings. And, when we look at that, that’s the portion we want to see more people have private insurance to, so that they don’t actually fall through the cracks of this. And, in Asia, specifically, there’s $1.8 trillion of required medical health care that people actually need, but they don’t actually end up getting because they don’t have enough savings or private health insurance to cover.
Shereen Abdulla
What does that stat represent, exactly?
Christina Cai
$1.8 trillion is the gap between how much health care people actually need, but they don’t get because they can’t pay for it.
Shereen Abdulla
So, $1.8 trillion in medical care?
Christina Cai
In medical care, yeah, that people need but they don’t get because they can’t pay for it. So that’s really the out-of-pocket expenses, those are things that people have to pay out-of-pocket, that they don’t have private health insurance to cover and they don’t have the necessary savings in order to be able to cover that amount.
Shereen Abdulla
Where’s that data from, by the way?
Christina Cai
Swiss Reinsurance.
Shereen Abdulla
Got that. Now, Christina, you’re based in Toronto and your co-founder’s based in Taiwan — why did you set up Lydia AI in Taiwan?
Christina Cai
So, we actually started out of the University of Toronto with a piece of machine learning technology. So, actually, our entire machine learning team is in Toronto and it’s actually our clients that recognize what our algos can do and actually evolved with us, it evolved with them into Asia and they said, “Hey, this is the region that you should really target. You’d be able to get traction and go to market really quickly to prove out that, ‘Hey, you can use your sources to underwrite,’ then expand into other areas.” So, at that point, when we had the technology, we started building out a go-to-market and sales and marketing team out in Taiwan. And now, we’re going to build one in Singapore, as well, to really expand first in that region, show the initial traction, show that the idea works, and then really see how we go to market.
Shereen Abdulla
You said that the initial problem that Lydia AI wanted to solve was particular to emerging markets, and Asia, specifically. But, why Taiwan?
Christina Cai
The reason we picked Taiwan is because they’re actually one of the leaders in what we call “open health data.” So, this is about data availability, and what we mean by that is that the government has actually invested heavily into creating this eHealth framework of which having the population’s electronic medical health records centralized as part of their universal health care. So, when you make a claim with the government, they actually store your health; and they wanted to make that open. So, they actually have a health API that allows customers to actually consent this type of data to trusted third parties. So, with Taiwan, it was a market that really — it’s actually the 10th largest insurance market, 3rd in Asia — so, really big insurance market. And also, there was a really friendly data environment, because the government is heavily invested into these initiatives, that we had the data environment we needed in order to quickly prove out the traction that we needed to see with a health risk score, to enable us to grow into other markets.
Shereen Abdulla
Can you describe the healthcare system in Taiwan?
Christina Cai
So, there is universal health care that covers basic health care expenditures, and then there are portions of it depending on specialty treatments (especially in the realm of cancer treatments) that are purely out-of-pocket. So, unlike Canada —where our R&D and machine learning team is in, where most things are actually covered under universal health care — in Taiwan, specifically, there are certain things that are covered under the government. Then, there are certain things that are covered in these out-of-pocket expenses. Usually, people buy private health insurance or use savings to cover that gap between the universal health coverage and the out-of-pocket expenses.
Shereen Abdulla
And how do these markets compare to other health systems?
Christina Cai
I think, out-of-pocket expenses — the combination of universal health care and out-of-pocket expenses and having some sort of insurance schemes, whether it’s government-mandated like in the US, or government-covered (because we have a single payer system in Canada, or in other areas), it’s really about how — what is the portion where people typically can expect to actually pay for, and do they have a way that they’ve planned early in order to pay for that. So, that’s really what we’re looking for.
Christina Cai
So, regardless of which country you’re in, it’s some system of universal basic universal health care, government will cover out-of-pocket expenses that needs insurance to cover — whether that’s through a government mandated plan, like in the US, or a single payer system, like in Canada, or in many countries across Asia — just private health insurance covered by the individual, because there’s no government mandate plan.
Shereen Abdulla
Who are Lydia AI’s customers?
Christina Cai
So, we sell at a B2B2C model. So, we actually work with insurance companies to validate the health risk score matched against insurance products. Then, from there on, there is a white label application that we give to the insurers who then actually give it to their customers. And that’s actually how we reach the 2C. So, our customers, our insurance companies, who then use our technology to serve customers.
Shereen Abdulla
And where do the brokers fit into this distribution model?
Christina Cai
So, in Asia, a lot of the times, online insurance sales only account for a very small portion of the market. So, most of insurance sales are actually still done by agents, either remotely via conference call or in-person. So, the way that it would work is that a — insurance companies would purchase our health risk score then distribute it to their broker/ agents. And it’s actually the on-the-ground agents, who then push it to the customers and actually use this health report as almost a shared health context to make the best recommendations to the customers and then lead them through the buying process.
Shereen Abdulla
And when it comes to working with insurance companies as your customers, what information exactly do you provide them? I know that you alluded to wearables and open health data at the beginning of the conversation.
Christina Cai
The way it works is customers will consent data, wearable data such as the step accelerometer, medical devices (such as glucose monitors or blood pressure monitors). Those are some of the metrics we would take — electronic medical records. We would then ingest it and actually produce a series of health risk scores, comparing the person with people in their age and gender group. Now, those risk scores are actually sent directly to the customers first, and customers get this personalized AI health report. They review it to get a better understanding of their health, and should they choose — they can choose a QR code that the agent scans in. And what the insurer actually gets is that they just get a green light, or a green light for all of the policies that the person can qualify for, to buy without a medical exam. So, they don’t actually get a person’s medical records or any of their health risk scores, they just get a how-does-this-person-actually-compare against pre-established thresholds that we’ve done with the insurer already.
Shereen Abdulla
What proportion of the data actually comes from wearables versus the eHealth online database? And I’m wondering, do people who not wear of wearables, are they at a disadvantage to being excluded from what Lydia AI does?
Christina Cai
No, because then we have — they can actually fill out questionnaires. So, the way that, like — our first iteration, it was just with electronic medical records, actually. And that’s because that’s the type of data that insurers were most familiar with to underwrite their policies. Then we started adding wearables on top of it. Insurers are starting to really get their feet wet with wearables, with the improvements of how they’re — good they are. They’re actually seeing that, “Hey, these risk assessments are getting much better than they were 2/ 5/ 10 years ago, that we can actually start considering them.” If they don’t have wearables and they don’t have health reports, then they can actually fill out a lifestyle questionnaire.
Shereen Abdulla
And how does Lydia AI validate the data that the consumer is inputting in the app?
Christina Cai
So, that will need a bit of back-checking, and you will need a secondary data source to do that. For example, if in lifestyle you say, “Hey, I exercise, you know, three times a day,” but your steps or your wearable tracker isn’t reflecting that, then that is a bit of a red flag for us. So, when we think about it, you might get a personalized health report just based on lifestyle data and you didn’t consent to anything else, you got a really good score; but for yourself, as a consumer, how useful is that for you? Then, on the side of the insurer, we would then work with them to say, “Okay, what kind of, essentially, data validation checks would you like us to do to get you comfortable around this health risks?” Or, if a consumer is just gave out lifestyle data with nothing to actually gut-check almost against it, are you still comfortable with that? If they say, “Hey, you know what, you’re not comfortable with that,” then the customer can still get their personalized health report based on whatever they inputted. What they wouldn’t get is personalized recommendations and pre-approval for certain products.
Shereen Abdulla
Having looked at the stats, how often do you find that people’s biological and chronological ages do, in fact, differ?
Christina Cai
Actually, quite a bit. And then, you know what, with an AI health report like this, it ends up happening that it — there is probably a positive selection here, where the people who want to actively seek out an AI health report are actually those who really care about their health. So, more often than not, we actually do see that their risks are a lot lower than the people in their age and gender group. And that’s because, when you look at it, like, you’re forced bell-curving it. And, you know what, the actuality is not bell-curved, as much as we would like to see that perfect shape of things.
Shereen Abdulla
Christina, you mentioned earlier that Lydia AI always obtains consent from the end consumers and that, in fact, they receive their health reports, first. How exactly do you get their consent, obtain this data, and then how are the reports shared back with them?
Christina Cai
Through the white label mobile app. So, we actually give out the mobile app so the customers — inside the customers ‘phone — and they actually get to pick: this is what I want to share, this is what I don’t want to share. Then, based on that — let’s say they share very little age — the lowest we can do is just, actually, gender and age — you’re not going to get anything descriptive, but they will get something back and they can say, “Wow, this is actually pretty cool. Maybe if I share a little bit more, I’d get a more precise and accurate one. Okay, if I share a little bit more —” So, it’s really about how do you create value for the end customer for the data that you’re — that they’re providing us with? And if customers actually find value in either an AI health report or getting personalized recommendations for this, and they’re willing to actually provide their data and do it — in our experience in Taiwan, over 90% of consumers have actually consented their data to us. So there’s two parts of it. So, customers consent to Lydia AI. Customers receive a personalized health AR report. So, that is the first part of it. And, the second part of it is between Lydia AI and the insurer. For us, it’s really important that we are maintaining the data privacy and data confidentiality of the customer, end-customer.
Shereen Abdulla
And how does all that, in turn, help make insurance more accessible for the end consumers?
Christina Cai
So, by two ways. The first way is it radically reduces the barrier to buying this type of health and life insurance. I don’t think anybody wants to go get a doc — wants to go get their blood drawn, which was necessary in the traditional way of risk assessment. So, by lowering the barrier of actually buying insurance, more people are going to buy it. So, the customer experience is much better, now — they have a much better understanding of it. And, the second portion, and this is what we’re really excited to at Lydia AI, is looking at people who were previously either rejected or ended up with a very high rate because of pre-existing diseases — we want to look at this group of underinsured, underserved people and say, “Based on new sources, you are actually healthy. Despite your pre-existing conditions, you’ve made healthy choices.”
Christina Cai
The best example of this is, actually, people with diabetes, who have very healthy lifestyles and ended up with very healthy futures. So, those are the types of people that we look at — these groups — and we say, “Okay, so we have the ability to actually select, out these groups, and let’s make sure this group of people are able to buy life and health insurance policies affordably.”
Shereen Abdulla
Christina, what have been some of the challenges you faced building Lydia AI?
Christina Cai
I think, one of the biggest challenges was finding our way to product-market fit. And, what I mean by that is — because we started out of school, we went the wrong way. We did technology to find a way into a problem, where this technology can make an impact, as opposed to you start it with the problem and then you found a way to solve this problem. So, we definitely went through this very roundabout.
Christina Cai
So, in this journey of actually working towards finding the impact of the technology, that was a really big challenge — actually trying to tune out where the signals were that noise, and was very much actually learning to speak to clients, asking them about their pain points, and actually trying to see whether or not there is a fit and being okay, when that door gets closed on your face.
Christina Cai
So, I’d say that was one of our biggest challenges — coming out of being first-time founders, coming to university with a piece of technology, targeting a behemoth industry like insurance. We’ve been very lucky to actually have insurance executives onboard our teams and actually give us that guidance and give us that expertise. But, starting out that journey of getting there was extremely difficult, but very glad we did it.
Shereen Abdulla
Christina, has regulation been an issue?
Christina Cai
So the way that we think about regulation — it hasn’t. The short answer is it hasn’t because we play within the regulations. It depends, market-to-market; but, really, we focus on trying to get insurance to more people. So, there are certain things that govern whether or not pricing can actually do it — we don’t touch that, we just focus on, “Okay, this is now thresholding better people.” Green light, red light. So, we actually look at — this is what the regulation allows, and this is how we’re going to play within that boundary in order to help our insurance companies actually get to market faster within the allowance of regulations.
Shereen Abdulla
You mentioned you do want to expand beyond Taiwan. With that in mind, how can you adapt to other markets, given there are different healthcare systems around the world?
Christina Cai
So, really, the way that I see, is we internationalized from day zero and now we’re facing the challenge of, “Okay, so how do we actually expand market-by-market-by-market-by-market.” Insurance is a heavily-regulated industry; so, it looks like, “Okay, in each market, what is the fastest way to actually show the traction.” We need to get to where we need to be. So, the way that we will adapt is we will look at data source availability first, then we will look at — okay, some markets that electronic medical records are just not available, in which case we will look at, okay, well, what about wearables (in terms of steps, in terms of sleep, in terms of all of these). If these are available, then we will match that against what are the needs; like, if this healthcare system looks like this and most of the out-of-pocket expenses are coming from this type of treatments for it, maybe it’s cancer, then we would then actually work with the insurance to look at — okay, given the data availability, here are the health risk scores we can produce, how does this match against your risk appetite? Because, you know what, insurance companies are actually the — they deal in risk. They’re the undertakers of all the risk. How does this match against your risk appetite in order for us to bring something to market? So that is the approach by which we would look at it, regardless of which healthcare system we’re in.
Shereen Abdulla
With that in mind, what’s next for Lydia AI?
Christina Cai
So, now that we’ve proven traction in Taiwan (that was our first market), we actually want to replicate the same model in Singapore, South Korea, and Japan. Those are the markets that we’re starting, and as we get more traction in these, we’ll be expanding to the rest of Southeast Asia, followed by Latin America, and then the Middle East region. So, for us, it’s really about how do we reach the next billion people and improve their life, health, and prosperity.
Shereen Abdulla
Alright, Christina, thank you very much for coming on the show was wonderful to chat to you.
Christina Cai
Thank you so much, appreciate the opportunity.
Shereen Abdulla
Absolutely.
LEGAL DISCLAIMER THIS PODCAST IS INTENDED SOLELY FOR GENERAL INFORMATIONAL OR EDUCATIONAL PURPOSES ONLY. UNDER NO CIRCUMSTANCES SHOULD ANY CONTENT PROVIDED AS PART OF ANY SUCH PROGRAMS, SERVICES OR EVENTS BE CONSTRUED AS INVESTMENT, LEGAL, TAX OR ACCOUNTING ADVICE BY 500 STARTUPS MANAGEMENT COMPANY, L.L.C. OR ANY OF ITS AFFILIATES (“500 GLOBAL”). 500 GLOBAL MAKES NO REPRESENTATION AS TO THE ACCURACY OR INFORMATION IN THIS PODCAST AND WHILE REASONABLE STEPS HAVE BEEN TAKEN TO ENSURE THAT THE INFORMATION HEREIN IS ACCURATE AND UP-TO-DATE, NO LIABILITY CAN BE ACCEPTED FOR ANY ERROR OR OMISSIONS AND 500 GLOBAL ACCEPTS NO RESPONSIBILITY FOR ANY LOSS WHICH MAY ARISE FROM RELIANCE ON THE INFORMATION IN THIS PODCAST. UNDER NO CIRCUMSTANCES SHOULD ANY INFORMATION OR CONTENT IN THIS PODCAST, BE CONSIDERED AS AN OFFER TO SELL OR SOLICITATION OF INTEREST TO PURCHASE ANY SECURITIES ADVISED BY 500 GLOBAL OR ANY OF ITS AFFILIATES OR REPRESENTATIVES. FURTHER, NO CONTENT OR INFORMATION IN THIS PODCAST IS INTENDED AS AN OFFER TO PROVIDE ANY INVESTMENT ADVISORY SERVICE WITH REGARD TO SECURITIES BY 500 GLOBAL. UNDER NO CIRCUMSTANCES SHOULD ANYTHING HEREIN BE CONSTRUED AS FUND MARKETING MATERIALS BY PROSPECTIVE INVESTORS CONSIDERING AN INVESTMENT INTO ANY 500 GLOBAL INVESTMENT FUND. UNDER NO CIRCUMSTANCES SHOULD ANY STATISTICS, QUOTATIONS OR OTHER CONTENT BE INTERPRETED AS TESTIMONIALS OR ENDORSEMENT OF THE INVESTMENT PERFORMANCE OF ANY 500 GLOBAL FUND BY A PROSPECTIVE INVESTOR CONSIDERING AN INVESTMENT INTO ANY 500 GLOBAL FUND. THIS PODCAST MAY CONTAIN FORWARD-LOOKING STATEMENTS WHICH INVOLVE RISKS AND UNCERTAINTIES, AND ACTUAL RESULTS MAY DIFFER MATERIALLY FROM ANY EXPECTATIONS, PROJECTIONS OR PREDICTIONS MADE OR IMPLICATED IN SUCH FORWARD-LOOKING STATEMENTS. THE PODCAST INCLUDES CONTENT DELIVERED BY AN INDEPENDENT THIRD PARTY THAT IS NOT RELATED TO OR CONTROLLED BY 500 GLOBAL. ALL VIEWS AND OPINIONS PRESENTED IN THE PODCAST BY SUCH THIRD PARTY ARE THEIR OWN VIEWS AND OPINIONS AND DO NOT REPRESENT THOSE OF 500 GLOBAL. 500 GLOBAL MAKES NO REPRESENTATIONS AS TO OR GUARANTEES OF SPECIFIC OUTCOMES FROM ATTENDING OR RELYING ON THE CONTENTS OF THE PODCAST.